Our group, led by Rajesh Ranganath, applies probabilistic approaches to tackle a wide range of fundamental challenges in machine learning. From the NYU Courant Institute of Mathematical Sciences (Computer Science), the NYU Center for Data Science, and NYU Langone Health, we focus on areas including but not limited to:
ai for healthcare and science
- QTNet: Predicting Drug-Induced QT Prolongation With Artificial Intelligence–Enabled ElectrocardiogramsHao Zhang, Constantine Tarabanis, Neil Jethani, Mark Goldstein, Silas Smith, Larry Chinitz, Rajesh Ranganath, Yindalon Aphinyanaphongs, and Lior Jankelson2024
Prediction of drug-induced long QT syndrome (diLQTS) is of critical importance given its association with torsades de pointes. There is no reliable method for the outpatient prediction of diLQTS. This study sought to evaluate the use of a convolutional neural network (CNN) applied to electrocardiograms (ECGs) to predict diLQTS in an outpatient population. We identified all adult outpatients newly prescribed a QT-prolonging medication between January 1, 2003, and March 31, 2022, who had a 12-lead sinus ECG in the preceding 6 months. Using risk factor data and the ECG signal as inputs, the CNN QTNet was implemented in TensorFlow to predict diLQTS. Models were evaluated in a held-out test dataset of 44,386 patients (57% female) with a median age of 62 years. Compared with 3 other models relying on risk factors or ECG signal or baseline QTc alone, QTNet achieved the best (P < 0.001) performance with a mean area under the curve of 0.802 (95% CI: 0.786-0.818). In a survival analysis, QTNet also had the highest inverse probability of censorship–weighted area under the receiver-operating characteristic curve at day 2 (0.875; 95% CI: 0.848-0.904) and up to 6 months. In a subgroup analysis, QTNet performed best among males and patients ≤50 years or with baseline QTc <450 ms. In an external validation cohort of solely suburban outpatient practices, QTNet similarly maintained the highest predictive performance. An ECG-based CNN can accurately predict diLQTS in the outpatient setting while maintaining its predictive performance over time. In the outpatient setting, our model could identify higher-risk individuals who would benefit from closer monitoring.
- Quantifying impairment and disease severity using AI models trained on healthy subjectsBoyang Yu, Aakash Kaku, Kangning Liu, Avinash Parnandi, Emily Fokas, Anita Venkatesan, Natasha Pandit, Rajesh Ranganath, Heidi Schambra, and Carlos Fernandez-Grandanpj Digital Medicine 2024
Automatic assessment of impairment and disease severity is a key challenge in data-driven medicine. We propose a framework to address this challenge, which leverages AI models trained exclusively on healthy individuals. The COnfidence-Based chaRacterization of Anomalies (COBRA) score exploits the decrease in confidence of these models when presented with impaired or diseased patients to quantify their deviation from the healthy population. We applied the COBRA score to address a key limitation of current clinical evaluation of upper-body impairment in stroke patients. The gold-standard Fugl-Meyer Assessment (FMA) requires in-person administration by a trained assessor for 30-45 minutes, which restricts monitoring frequency and precludes physicians from adapting rehabilitation protocols to the progress of each patient. The COBRA score, computed automatically in under one minute, is shown to be strongly correlated with the FMA on an independent test cohort for two different data modalities: wearable sensors (ρ = 0.814, 95% CI [0.700,0.888]) and video (ρ = 0.736, 95% C.I [0.584, 0.838]). To demonstrate the generalizability of the approach to other conditions, the COBRA score was also applied to quantify severity of knee osteoarthritis from magnetic-resonance imaging scans, again achieving significant correlation with an independent clinical assessment (ρ = 0.644, 95% C.I [0.585,0.696]).
- Robust Anomaly Detection for Particle Physics Using Multi-background Representation LearningAbhijith Gandrakota, Lily H. Zhang, Aahlad Puli, Kyle Cranmer, Jennifer Ngadiuba, Rajesh Ranganath, and Nhan TranMLST 2024
Anomaly, or out-of-distribution, detection is a promising tool for aiding discoveries of new particles or processes in particle physics. In this work, we identify and address two overlooked opportunities to improve anomaly detection for high-energy physics. First, rather than train a generative model on the single most dominant background process, we build detection algorithms using representation learning from multiple background types, thus taking advantage of more information to improve estimation of what is relevant for detection. Second, we generalize decorrelation to the multi-background setting, thus directly enforcing a more complete definition of robustness for anomaly detection. We demonstrate the benefit of the proposed robust multi-background anomaly detection algorithms on a high-dimensional dataset of particle decays at the Large Hadron Collider.
- Adaptive Sampling of k-Space in Magnetic Resonance for Rapid Pathology PredictionChen-Yu Yen, Raghav Singhal, Umang Sharma, Rajesh Ranganath, Sumit Chopra, and Lerrel PintoICML 2024
Magnetic Resonance (MR) imaging, despite its proven diagnostic utility, remains an inaccessible imaging modality for disease surveillance at the population level. A major factor rendering MR inaccessible is lengthy scan times. An MR scanner collects measurements associated with the underlying anatomy in the Fourier space, also known as the k-space. Creating a high-fidelity image requires collecting large quantities of such measurements, increasing the scan time. Traditionally to accelerate an MR scan, image reconstruction from under-sampled k-space data is the method of choice. However, recent works show the feasibility of bypassing image reconstruction and directly learning to detect disease directly from a sparser learned subset of the k-space measurements. In this work, we propose Adaptive Sampling for MR (ASMR), a sampling method that learns an adaptive policy to sequentially select k-space samples to optimize for target disease detection. On 6 out of 8 pathology classification tasks spanning the Knee, Brain, and Prostate MR scans, ASMR reaches within 2% of the performance of a fully sampled classifier while using only 8% of the k-space, as well as outperforming prior state-of-the-art work in k-space sampling such as EMRT, LOUPE, and DPS.
- Causal inference in oncology: why, what, how and whenWouter AC Amsterdam, Sjoerd Elias, and Rajesh RanganathClinical Oncology 2024
Oncologists are faced with choosing the best treatment for each patient, based on the available evidence from randomized controlled trials (RCTs) and observational studies. RCTs provide estimates of the average effects of treatments on groups of patients, but they may not apply in many real-world scenarios where for example patients have different characteristics than the RCT participants, or where different treatment variants are considered. Causal inference defines what a treatment effect is and how it may be estimated with RCTs or outside of RCTs with observational – or ‘real-world’ – data. In this review, we introduce the field of causal inference, explain what a treatment effect is and what important challenges are with treatment effect estimation with observational data. We then provide a framework for conducting causal inference studies and describe when in oncology causal inference from observational data may be particularly valuable. Recognizing the strengths and limitations of both RCTs and observational causal inference provides a way for more informed and individualized treatment decision-making in oncology.
- From algorithms to action: improving patient care requires causalityWouter AC Amsterdam, Pim A Jong, Joost JC Verhoeff, Tim Leiner, and Rajesh RanganathBMC medical informatics and decision making 2024
In cancer research there is much interest in building and validating outcome prediction models to support treatment decisions. However, because most outcome prediction models are developed and validated without regard to the causal aspects of treatment decision making, many published outcome prediction models may cause harm when used for decision making, despite being found accurate in validation studies. Guidelines on prediction model validation and the checklist for risk model endorsement by the American Joint Committee on Cancer do not protect against prediction models that are accurate during development and validation but harmful when used for decision making. We explain why this is the case and how to build and validate models that are useful for decision making.
- When accurate prediction models yield harmful self-fulfilling propheciesWouter AC Amsterdam, Nan Geloven, Jesse H Krijthe, Rajesh Ranganath, and Giovanni Ciná2023
Prediction models are popular in medical research and practice. By predicting an outcome of interest for specific patients, these models may help inform difficult treatment decisions, and are often hailed as the poster children for personalized, data-driven healthcare. We show however, that using prediction models for decision making can lead to harmful decisions, even when the predictions exhibit good discrimination after deployment. These models are harmful self-fulfilling prophecies: their deployment harms a group of patients but the worse outcome of these patients does not invalidate the predictive power of the model. Our main result is a formal characterization of a set of such prediction models. Next we show that models that are well calibrated before and after deployment are useless for decision making as they made no change in the data distribution. These results point to the need to revise standard practices for validation, deployment and evaluation of prediction models that are used in medical decisions.
- Conditional average treatment effect estimation with marginally constrained modelsWouter AC Van Amsterdam, and Rajesh RanganathJournal of Causal Inference 2023
Treatment effect estimates are often available from randomized controlled trials as a single average treatment effect for a certain patient population. Estimates of the conditional average treatment effect (CATE) are more useful for individualized treatment decision-making, but randomized trials are often too small to estimate the CATE. Examples in medical literature make use of the relative treatment effect (e.g. an odds ratio) reported by randomized trials to estimate the CATE using large observational datasets. One approach to estimating these CATE models is by using the relative treatment effect as an offset, while estimating the covariate-specific untreated risk. We observe that the odds ratios reported in randomized controlled trials are not the odds ratios that are needed in offset models because trials often report the marginal odds ratio. We introduce a constraint or a regularizer to better use marginal odds ratios from randomized controlled trials and find that under the standard observational causal inference assumptions, this approach provides a consistent estimate of the CATE. Next, we show that the offset approach is not valid for CATE estimation in the presence of unobserved confounding. We study if the offset assumption and the marginal constraint lead to better approximations of the CATE relative to the alternative of using the average treatment effect estimate from the randomized trial. We empirically show that when the underlying CATE has sufficient variation, the constraint and offset approaches lead to closer approximations to the CATE.
- Individual treatment effect estimation in the presence of unobserved confounding using proxies: a cohort study in stage III non-small cell lung cancerWouter AC Amsterdam, Joost JC Verhoeff, Netanja I Harlianto, Gijs A Bartholomeus, Aahlad Manas Puli, Pim A Jong, Tim Leiner, Anne SR Lindert, Marinus JC Eijkemans, and Rajesh RanganathScientific reports 2022
Randomized Controlled Trials (RCT) are the gold standard for estimating treatment effects but some important situations in cancer care require treatment effect estimates from observational data. We developed “Proxy based individual treatment effect modeling in cancer” (PROTECT) to estimate treatment effects from observational data when there are unobserved confounders, but proxy measurements of these confounders exist. We identified an unobserved confounder in observational cancer research: overall fitness. Proxy measurements of overall fitness exist like performance score, but the fitness as observed by the treating physician is unavailable for research. PROTECT reconstructs the distribution of the unobserved confounder based on these proxy measurements to estimate the treatment effect. PROTECT was applied to an observational cohort of 504 stage III non-small cell lung cancer (NSCLC) patients, treated with concurrent chemoradiation or sequential chemoradiation. Whereas conventional confounding adjustment methods seemed to overestimate the treatment effect, PROTECT provided credible treatment effect estimates.
- Probabilistic machine learning for healthcareIrene Y Chen, Shalmali Joshi, Marzyeh Ghassemi, and Rajesh RanganathAnnual review of biomedical data science 2021
Machine learning can be used to make sense of healthcare data. Probabilistic machine learning models help provide a complete picture of observed data in healthcare. In this review, we examine how probabilistic machine learning can advance healthcare. We consider challenges in the predictive model building pipeline where probabilistic models can be beneficial including calibration and missing data. Beyond predictive models, we also investigate the utility of probabilistic machine learning models in phenotyping, in generative models for clinical use cases, and in reinforcement learning.
- Deep learning models for electrocardiograms are susceptible to adversarial attackXintian Han, Yuxuan Hu, Luca Foschini, Larry Chinitz, Lior Jankelson, and Rajesh RanganathNature Medicine 2020
Electrocardiogram (ECG) acquisition is increasingly widespread in medical and commercial devices, necessitating the development of automated interpretation strategies. Recently, deep neural networks have been used to automatically analyze ECG tracings and outperform physicians in detecting certain rhythm irregularities. However, deep learning classifiers are susceptible to adversarial examples, which are created from raw data to fool the classifier such that it assigns the example to the wrong class, but which are undetectable to the human eye. Adversarial examples have also been created for medical-related tasks. However, traditional attack methods to create adversarial examples do not extend directly to ECG signals, as such methods introduce square-wave artefacts that are not physiologically plausible. Here we develop a method to construct smoothed adversarial examples for ECG tracings that are invisible to human expert evaluation and show that a deep learning model for arrhythmia detection from single-lead ECG is vulnerable to this type of attack. Moreover, we provide a general technique for collating and perturbing known adversarial examples to create multiple new ones. The susceptibility of deep learning ECG algorithms to adversarial misclassification implies that care should be taken when evaluating these models on ECGs that may have been altered, particularly when incentives for causing misclassification exist.
- A validated, real-time prediction model for favorable outcomes in hospitalized COVID-19 patientsNarges Razavian, Vincent J. Major, Mukund Sudarshan, Jesse Burk-Rafel, Peter Stella, Hardev Randhawa, Seda Bilaloglu, Ji Chen, Vuthy Nguy, Walter Wang, Hao Zhang, Ilan Reinstein, David Kudlowitz, Cameron Zenger, Meng Cao, Ruina Zhang, Siddhant Dogra, Keerthi B. Harish, Brian Bosworth, Fritz Francois, Leora I. Horwitz, Rajesh Ranganath, Jonathan Austrian, and Yindalon Aphinyanaphongs2020
The COVID-19 pandemic has challenged front-line clinical decision-making, leading to numerous published prognostic tools. However, few models have been prospectively validated and none report implementation in practice. Here, we use 3345 retrospective and 474 prospective hospitalizations to develop and validate a parsimonious model to identify patients with favorable outcomes within 96 h of a prediction, based on real-time lab values, vital signs, and oxygen support variables. In retrospective and prospective validation, the model achieves high average precision (88.6% 95% CI: [88.4–88.7] and 90.8% [90.8–90.8]) and discrimination (95.1% [95.1–95.2] and 86.8% [86.8–86.9]) respectively. We implemented and integrated the model into the EHR, achieving a positive predictive value of 93.3% with 41% sensitivity. Preliminary results suggest clinicians are adopting these scores into their clinical workflows.
- Clinicalbert: Modeling clinical notes and predicting hospital readmissionKexin Huang, Jaan Altosaar, and Rajesh Ranganath2019
Clinical notes contain information about patients that goes beyond structured data like lab values and medications. However, clinical notes have been underused relative to structured data, because notes are high-dimensional and sparse. This work develops and evaluates representations of clinical notes using bidirectional transformers (ClinicalBERT). ClinicalBERT uncovers high-quality relationships between medical concepts as judged by humans. ClinicalBert outperforms baselines on 30-day hospital readmission prediction using both discharge summaries and the first few days of notes in the intensive care unit. Code and model parameters are available.
causal inference and decision making
- Causal inference in oncology: why, what, how and whenWouter AC Amsterdam, Sjoerd Elias, and Rajesh RanganathClinical Oncology 2024
Oncologists are faced with choosing the best treatment for each patient, based on the available evidence from randomized controlled trials (RCTs) and observational studies. RCTs provide estimates of the average effects of treatments on groups of patients, but they may not apply in many real-world scenarios where for example patients have different characteristics than the RCT participants, or where different treatment variants are considered. Causal inference defines what a treatment effect is and how it may be estimated with RCTs or outside of RCTs with observational – or ‘real-world’ – data. In this review, we introduce the field of causal inference, explain what a treatment effect is and what important challenges are with treatment effect estimation with observational data. We then provide a framework for conducting causal inference studies and describe when in oncology causal inference from observational data may be particularly valuable. Recognizing the strengths and limitations of both RCTs and observational causal inference provides a way for more informed and individualized treatment decision-making in oncology.
- From algorithms to action: improving patient care requires causalityWouter AC Amsterdam, Pim A Jong, Joost JC Verhoeff, Tim Leiner, and Rajesh RanganathBMC medical informatics and decision making 2024
In cancer research there is much interest in building and validating outcome prediction models to support treatment decisions. However, because most outcome prediction models are developed and validated without regard to the causal aspects of treatment decision making, many published outcome prediction models may cause harm when used for decision making, despite being found accurate in validation studies. Guidelines on prediction model validation and the checklist for risk model endorsement by the American Joint Committee on Cancer do not protect against prediction models that are accurate during development and validation but harmful when used for decision making. We explain why this is the case and how to build and validate models that are useful for decision making.
- When accurate prediction models yield harmful self-fulfilling propheciesWouter AC Amsterdam, Nan Geloven, Jesse H Krijthe, Rajesh Ranganath, and Giovanni Ciná2023
Prediction models are popular in medical research and practice. By predicting an outcome of interest for specific patients, these models may help inform difficult treatment decisions, and are often hailed as the poster children for personalized, data-driven healthcare. We show however, that using prediction models for decision making can lead to harmful decisions, even when the predictions exhibit good discrimination after deployment. These models are harmful self-fulfilling prophecies: their deployment harms a group of patients but the worse outcome of these patients does not invalidate the predictive power of the model. Our main result is a formal characterization of a set of such prediction models. Next we show that models that are well calibrated before and after deployment are useless for decision making as they made no change in the data distribution. These results point to the need to revise standard practices for validation, deployment and evaluation of prediction models that are used in medical decisions.
- Conditional average treatment effect estimation with marginally constrained modelsWouter AC Van Amsterdam, and Rajesh RanganathJournal of Causal Inference 2023
Treatment effect estimates are often available from randomized controlled trials as a single average treatment effect for a certain patient population. Estimates of the conditional average treatment effect (CATE) are more useful for individualized treatment decision-making, but randomized trials are often too small to estimate the CATE. Examples in medical literature make use of the relative treatment effect (e.g. an odds ratio) reported by randomized trials to estimate the CATE using large observational datasets. One approach to estimating these CATE models is by using the relative treatment effect as an offset, while estimating the covariate-specific untreated risk. We observe that the odds ratios reported in randomized controlled trials are not the odds ratios that are needed in offset models because trials often report the marginal odds ratio. We introduce a constraint or a regularizer to better use marginal odds ratios from randomized controlled trials and find that under the standard observational causal inference assumptions, this approach provides a consistent estimate of the CATE. Next, we show that the offset approach is not valid for CATE estimation in the presence of unobserved confounding. We study if the offset assumption and the marginal constraint lead to better approximations of the CATE relative to the alternative of using the average treatment effect estimate from the randomized trial. We empirically show that when the underlying CATE has sufficient variation, the constraint and offset approaches lead to closer approximations to the CATE.
- Individual treatment effect estimation in the presence of unobserved confounding using proxies: a cohort study in stage III non-small cell lung cancerWouter AC Amsterdam, Joost JC Verhoeff, Netanja I Harlianto, Gijs A Bartholomeus, Aahlad Manas Puli, Pim A Jong, Tim Leiner, Anne SR Lindert, Marinus JC Eijkemans, and Rajesh RanganathScientific reports 2022
Randomized Controlled Trials (RCT) are the gold standard for estimating treatment effects but some important situations in cancer care require treatment effect estimates from observational data. We developed “Proxy based individual treatment effect modeling in cancer” (PROTECT) to estimate treatment effects from observational data when there are unobserved confounders, but proxy measurements of these confounders exist. We identified an unobserved confounder in observational cancer research: overall fitness. Proxy measurements of overall fitness exist like performance score, but the fitness as observed by the treating physician is unavailable for research. PROTECT reconstructs the distribution of the unobserved confounder based on these proxy measurements to estimate the treatment effect. PROTECT was applied to an observational cohort of 504 stage III non-small cell lung cancer (NSCLC) patients, treated with concurrent chemoradiation or sequential chemoradiation. Whereas conventional confounding adjustment methods seemed to overestimate the treatment effect, PROTECT provided credible treatment effect estimates.
- Offline rl without off-policy evaluationDavid Brandfonbrener, Will Whitney, Rajesh Ranganath, and Joan BrunaNeurIPS 2021
Most prior approaches to offline reinforcement learning (RL) have taken an iterative actor-critic approach involving off-policy evaluation. In this paper we show that simply doing one step of constrained/regularized policy improvement using an on-policy Q estimate of the behavior policy performs surprisingly well. This one-step algorithm beats the previously reported results of iterative algorithms on a large portion of the D4RL benchmark. The one-step baseline achieves this strong performance while being notably simpler and more robust to hyperparameters than previously proposed iterative algorithms. We argue that the relatively poor performance of iterative approaches is a result of the high variance inherent in doing off-policy evaluation and magnified by the repeated optimization of policies against those estimates. In addition, we hypothesize that the strong performance of the one-step algorithm is due to a combination of favorable structure in the environment and behavior policy.
- Offline contextual bandits with overparameterized modelsDavid Brandfonbrener, William Whitney, Rajesh Ranganath, and Joan BrunaICML 2021
Recent results in supervised learning suggest that while overparameterized models have the capacity to overfit, they in fact generalize quite well. We ask whether the same phenomenon occurs for offline contextual bandits. Our results are mixed. Value-based algorithms benefit from the same generalization behavior as overparameterized supervised learning, but policy-based algorithms do not. We show that this discrepancy is due to the \emphaction-stability of their objectives. An objective is action-stable if there exists a prediction (action-value vector or action distribution) which is optimal no matter which action is observed. While value-based objectives are action-stable, policy-based objectives are unstable. We formally prove upper bounds on the regret of overparameterized value-based learning and lower bounds on the regret for policy-based algorithms. In our experiments with large neural networks, this gap between action-stable value-based objectives and unstable policy-based objectives leads to significant performance differences.
- General control functions for causal effect estimation from ivsAahlad Puli, and Rajesh RanganathAdvances in neural information processing systems 2020
Causal effect estimation relies on separating the variation in the outcome into parts due to the treatment and due to the confounders. To achieve this separation, practitioners often use external sources of randomness that only influence the treatment called instrumental variables (IVs). We study variables constructed from treatment and IV that help estimate effects, called control functions. We characterize general control functions for effect estimation in a meta-identification result. Then, we show that structural assumptions on the treatment process allow the construction of general control functions, thereby guaranteeing identification. To construct general control functions and estimate effects, we develop the general control function method (GCFN). GCFN’s first stage called variational decoupling (VDE) constructs general control functions by recovering the residual variation in the treatment given the IV. Using VDE’s control function, GCFN’s second stage estimates effects via regression. Further, we develop semi-supervised GCFN to construct general control functions using subsets of data that have both IV and confounders observed as supervision; this needs no structural treatment process assumptions. We evaluate GCFN on low and high dimensional simulated data and on recovering the causal effect of slave export on modern community trust [30]
- Causal estimation with functional confoundersAahlad Puli, Adler Perotte, and Rajesh RanganathAdvances in neural information processing systems 2020
Causal inference relies on two fundamental assumptions: ignorability and positivity. We study causal inference when the true confounder value can be expressed as a function of the observed data; we call this setting estimation with functional confounders (EFC). In this setting ignorability is satisfied, however positivity is violated, and causal inference is impossible in general. We consider two scenarios where causal effects are estimable. First, we discuss interventions on a part of the treatment called functional interventions and a sufficient condition for effect estimation of these interventions called functional positivity. Second, we develop conditions for nonparametric effect estimation based on the gradient fields of the functional confounder and the true outcome function. To estimate effects under these conditions, we develop Level-set Orthogonal Descent Estimation (LODE). Further, we prove error bounds on LODE’s effect estimates, evaluate our methods on simulated and real data, and empirically demonstrate the value of EFC.
generative modeling
- Improving Large Language Models with Targeted Negative TrainingLily H. Zhang, Rajesh Ranganath, and Arya TafviziTMLR 2024
Generative models of language exhibit impressive capabilities but still place non-negligible probability mass over undesirable outputs. In this work, we address the task of updating a model to avoid unwanted outputs while minimally changing model behavior otherwise, a challenge we refer to as a minimal targeted update. We first formalize the notion of a minimal targeted update and propose a method to achieve such updates using negative examples from a model’s generations. Our proposed Targeted Negative Training (TNT) results in updates that keep the new distribution close to the original, unlike existing losses for negative signal which push down probability but do not control what the updated distribution will be. In experiments, we demonstrate that TNT yields a better trade-off between reducing unwanted behavior and maintaining model generation behavior than baselines, paving the way towards a modeling paradigm based on iterative training updates that constrain models from generating undesirable outputs while preserving their impressive capabilities.
- Preference Learning Algorithms do not Learn Preference RankingsAngelica Chen, Sadhika Malladi, Lily H. Zhang, Xinyi Chen, Qiuyi Zhang, Rajesh Ranganath, and Kyunghyun ChoNeurIPS 2024
Preference learning algorithms (e.g., RLHF and DPO) are frequently used to steer LLMs to produce generations that are more preferred by humans, but our understanding of their inner workings is still limited. In this work, we study the conventional wisdom that preference learning trains models to assign higher likelihoods to more preferred outputs than less preferred outputs, measured via ranking accuracy. Surprisingly, we find that most state-of-the-art preference-tuned models achieve a ranking accuracy of less than 60% on common preference datasets. We furthermore derive the idealized ranking accuracy that a preference-tuned LLM would achieve if it optimized the DPO or RLHF objective perfectly. We demonstrate that existing models exhibit a significant alignment gap – i.e., a gap between the observed and idealized ranking accuracies. We attribute this discrepancy to the DPO objective, which is empirically and theoretically ill-suited to fix even mild ranking errors in the reference model, and derive a simple and efficient formula for quantifying the difficulty of learning a given preference datapoint. Finally, we demonstrate that ranking accuracy strongly correlates with the empirically popular win rate metric when the model is close to the reference model used in the objective, shedding further light on the differences between on-policy (e.g., RLHF) and off-policy (e.g., DPO) preference learning algorithms.
- What’s the score? Automated Denoising Score Matching for Nonlinear DiffusionsRaghav Singhal, Mark Goldstein, and Rajesh RanganathICML 2024
Reversing a diffusion process by learning its score forms the heart of diffusion-based generative modeling and for estimating properties of scientific systems. The diffusion processes that are tractable center on linear processes with a Gaussian stationary distribution. This limits the kinds of models that can be built to those that target a Gaussian prior or more generally limits the kinds of problems that can be generically solved to those that have conditionally linear score functions. In this work, we introduce a family of tractable denoising score matching objectives, called local-DSM, built using local increments of the diffusion process. We show how local-DSM melded with Taylor expansions enables automated training and score estimation with nonlinear diffusion processes. To demonstrate these ideas, we use automated-DSM to train generative models using non-Gaussian priors on challenging low dimensional distributions and the CIFAR10 image dataset. Additionally, we use the automated-DSM to learn the scores for nonlinear processes studied in statistical physics.
- Stochastic interpolants with data-dependent couplingsMichael S Albergo, Mark Goldstein, Nicholas M Boffi, Rajesh Ranganath, and Eric Vanden-EijndenICML 2024 (spotlight)
spotlight
Generative models inspired by dynamical transport of measure – such as flows and diffusions – construct a continuous-time map between two probability densities. Conventionally, one of these is the target density, only accessible through samples, while the other is taken as a simple base density that is data-agnostic. In this work, using the framework of stochastic interpolants, we formalize how to \textitcouple the base and the target densities, whereby samples from the base are computed conditionally given samples from the target in a way that is different from (but does preclude) incorporating information about class labels or continuous embeddings. This enables us to construct dynamical transport maps that serve as conditional generative models. We show that these transport maps can be learned by solving a simple square loss regression problem analogous to the standard independent setting. We demonstrate the usefulness of constructing dependent couplings in practice through experiments in super-resolution and in-painting.
- Where to diffuse, how to diffuse, and how to get back: Automated learning for multivariate diffusionsRaghav Singhal, Mark Goldstein, and Rajesh RanganathICLR 2023
Diffusion-based generative models (DBGMs) perturb data to a target noise distribution and reverse this process to generate samples. The choice of noising process, or inference diffusion process, affects both likelihoods and sample quality. For example, extending the inference process with auxiliary variables leads to improved sample quality. While there are many such multivariate diffusions to explore, each new one requires significant model-specific analysis, hindering rapid prototyping and evaluation. In this work, we study Multivariate Diffusion Models (MDMs). For any number of auxiliary variables, we provide a recipe for maximizing a lower-bound on the MDMs likelihood without requiring any model-specific analysis. We then demonstrate how to parameterize the diffusion for a specified target noise distribution; these two points together enable optimizing the inference diffusion process. Optimizing the diffusion expands easy experimentation from just a few well-known processes to an automatic search over all linear diffusions. To demonstrate these ideas, we introduce two new specific diffusions as well as learn a diffusion process on the MNIST, CIFAR10, and ImageNet32 datasets. We show learned MDMs match or surpass bits-per-dims (BPDs) relative to fixed choices of diffusions for a given dataset and model architecture.
interpretability
- Explanations that reveal all through the definition of encodingAahlad Manas Puli, Nhi Nguyen, and Rajesh Ranganath2024
Feature attributions attempt to highlight what inputs drive predictive power. Good attributions or explanations are thus those that produce inputs that retain this predictive power; accordingly, evaluations of explanations score their quality of prediction. However, evaluations produce scores better than what appears possible from the values in the explanation for a class of explanations, called encoding explanations. Probing for encoding remains a challenge because there is no general characterization of what gives the extra predictive power. We develop a definition of encoding that identifies this extra predictive power via conditional dependence and show that the definition fits existing examples of encoding. This definition implies, in contrast to encoding explanations, that non-encoding explanations contain all the informative inputs used to produce the explanation, giving them a "what you see is what you get" property, which makes them transparent and simple to use. Next, we prove that existing scores (ROAR, FRESH, EVAL-X) do not rank non-encoding explanations above encoding ones, and develop STRIPE-X which ranks them correctly. After empirically demonstrating the theoretical insights, we use STRIPE-X to uncover encoding in LLM-generated explanations for predicting the sentiment in movie reviews.
- DIET: Conditional independence testing with marginal dependence measures of residual informationMukund Sudarshan, Aahlad Puli, Wesley Tansey, and Rajesh RanganathInternational Conference on Artificial Intelligence and Statistics 2023
Conditional randomization tests (CRTs) assess whether a variable is predictive of another variable , having observed covariates . CRTs require fitting a large number of predictive models, which is often computationally intractable. Existing solutions to reduce the cost of CRTs typically split the dataset into a train and test portion, or rely on heuristics for interactions, both of which lead to a loss in power. We propose the decoupled independence test (DIET), an algorithm that avoids both of these issues by leveraging marginal independence statistics to test conditional independence relationships. DIET tests the marginal independence of two random variables: and where is a conditional cumulative distribution function (CDF) for the distribution . These variables are termed “information residuals.” We give sufficient conditions for DIET to achieve finite sample type-1 error control and power greater than the type-1 error rate. We then prove that when using the mutual information between the information residuals as a test statistic, DIET yields the most powerful conditionally valid test. Finally, we show DIET achieves higher power than other tractable CRTs on several synthetic and real benchmarks.
- Don’t be fooled: label leakage in explanation methods and the importance of their quantitative evaluationNeil Jethani, Adriel Saporta, and Rajesh RanganathAISTATS 2023 (notable paper, oral presentation)
notable paper, oral presentation
Feature attribution methods identify which features of an input most influence a model’s output. Most widely-used feature attribution methods (such as SHAP, LIME, and Grad-CAM) are "class-dependent" methods in that they generate a feature attribution vector as a function of class. In this work, we demonstrate that class-dependent methods can "leak" information about the selected class, making that class appear more likely than it is. Thus, an end user runs the risk of drawing false conclusions when interpreting an explanation generated by a class-dependent method. In contrast, we introduce "distribution-aware" methods, which favor explanations that keep the label’s distribution close to its distribution given all features of the input. We introduce SHAP-KL and FastSHAP-KL, two baseline distribution-aware methods that compute Shapley values. Finally, we perform a comprehensive evaluation of seven class-dependent and three distribution-aware methods on three clinical datasets of different high-dimensional data types: images, biosignals, and text.
- FastSHAP: Real-Time Shapley Value EstimationNeil Jethani, Mukund Sudarshan, Ian Covert, Su-in Lee, and Rajesh RanganathICLR 2022 2022
- Contra: Contrarian statistics for controlled variable selectionMukund Sudarshan, Aahlad Puli, Lakshmi Subramanian, Sriram Sankararaman, and Rajesh RanganathInternational conference on artificial intelligence and statistics 2021
The holdout randomization test (HRT) discovers a set of covariates most predictive of a response. Given the covariate distribution, HRTs can explicitly control the false discovery rate (FDR). However, if this distribution is unknown and must be estimated from data, HRTs can inflate the FDR. To alleviate the inflation of FDR, we propose the contrarian randomization test (CONTRA), which is designed explicitly for scenarios where the covariate distribution must be estimated from data and may even be misspecified. Our key insight is to use an equal mixture of two “contrarian” probabilistic models in determining the importance of a covariate. One model is fit with the real data, while the other is fit using the same data, but with the covariate being tested replaced with samples from an estimate of the covariate distribution. CONTRA is flexible enough to achieve a power of 1 asymptotically, can reduce the FDR compared to state-of-the-art CVS methods when the covariate distribution is misspecified, and is computationally efficient in high dimensions and large sample sizes. We further demonstrate the effectiveness of CONTRA on numerous synthetic benchmarks, and highlight its capabilities on a genetic dataset.
- Have We Learned to Explain?: How Interpretability Methods Can Learn to Encode Predictions in their Interpretations.Neil Jethani, Mukund Sudarshan, Yindalon Aphinyanaphongs, and Rajesh RanganathAISTATS 2021
While the need for interpretable machine learning has been established, many common approaches are slow, lack fidelity, or hard to evaluate. Amortized explanation methods reduce the cost of providing interpretations by learning a global selector model that returns feature importances for a single instance of data. The selector model is trained to optimize the fidelity of the interpretations, as evaluated by a predictor model for the target. Popular methods learn the selector and predictor model in concert, which we show allows predictions to be encoded within interpretations. We introduce EVAL-X as a method to quantitatively evaluate interpretations and REAL-X as an amortized explanation method, which learn a predictor model that approximates the true data generating distribution given any subset of the input. We show EVAL-X can detect when predictions are encoded in interpretations and show the advantages of REAL-X through quantitative and radiologist evaluation.
neural networks and representation learning
- Contrasting with Symile: Simple Model-Agnostic Representation Learning for Unlimited ModalitiesAdriel Saporta, Aahlad Puli, Mark Goldstein, and Rajesh RanganathNeurIPS 2024
Contrastive learning methods, such as CLIP, leverage naturally paired data—for example, images and their corresponding text captions—to learn general representations that transfer efficiently to downstream tasks. While such approaches are generally applied to two modalities, domains such as robotics, healthcare, and video need to support many types of data at once. We show that the pairwise application of CLIP fails to capture joint information between modalities, thereby limiting the quality of the learned representations. To address this issue, we present Symile, a simple contrastive learning approach that captures higher-order information between any number of modalities. Symile provides a flexible, architecture-agnostic objective for learning modality-specific representations. To develop Symile’s objective, we derive a lower bound on total correlation, and show that Symile representations for any set of modalities form a sufficient statistic for predicting the remaining modalities. Symile outperforms pairwise CLIP, even with modalities missing in the data, on cross-modal classification and retrieval across several experiments including on an original multilingual dataset of 33M image, text and audio samples and a clinical dataset of chest X-rays, electrocardiograms, and laboratory measurements. All datasets and code used in this work are publicly available at https://github.com/rajesh-lab/symile.
- Set Norm and Equivariant Residual Connections: Putting the Deep in Deep SetsLily H. Zhang, Veronica Tozzo, John Higgins, and Rajesh RanganathICML 2022
Permutation invariant neural networks are a promising tool for predictive modeling of set data. We show, however, that existing architectures struggle to perform well when they are deep. In this work, we mathematically and empirically analyze normalization layers and residual connections in the context of deep permutation invariant neural networks. We develop set norm, a normalization tailored for sets, and introduce the “clean path principle” for equivariant residual connections alongside a novel benefit of such connections, the reduction of information loss. Based on our analysis, we propose Deep Sets++ and Set Transformer++, deep models that reach comparable or better performance than their original counterparts on a diverse suite of tasks. We additionally introduce Flow-RBC, a new single-cell dataset and real-world application of permutation invariant prediction. We open-source our data and code here: https://github.com/rajesh-lab/deep_permutation_invariant.
- Learning invariant representations with missing dataMark Goldstein, Jörn-Henrik Jacobsen, Olina Chau, Adriel Saporta, Aahlad Manas Puli, Rajesh Ranganath, and Andrew MillerConference on Causal Learning and Reasoning 2022
Spurious correlations allow flexible models to predict well during training but poorly on related test distributions. Recent work has shown that models that satisfy particular independencies involving correlation-inducing nuisance variables have guarantees on their test performance. Enforcing such independencies requires nuisances to be observed during training. However, nuisances, such as demographics or image background labels, are often missing. Enforcing independence on just the observed data does not imply independence on the entire population. Here we derive MMD estimators used for invariance objectives under missing nuisances. On simulations and clinical data, optimizing through these estimates achieves test performance similar to using estimators that make use of the full data.
out-of-distribution detection
- Quantifying impairment and disease severity using AI models trained on healthy subjectsBoyang Yu, Aakash Kaku, Kangning Liu, Avinash Parnandi, Emily Fokas, Anita Venkatesan, Natasha Pandit, Rajesh Ranganath, Heidi Schambra, and Carlos Fernandez-Grandanpj Digital Medicine 2024
Automatic assessment of impairment and disease severity is a key challenge in data-driven medicine. We propose a framework to address this challenge, which leverages AI models trained exclusively on healthy individuals. The COnfidence-Based chaRacterization of Anomalies (COBRA) score exploits the decrease in confidence of these models when presented with impaired or diseased patients to quantify their deviation from the healthy population. We applied the COBRA score to address a key limitation of current clinical evaluation of upper-body impairment in stroke patients. The gold-standard Fugl-Meyer Assessment (FMA) requires in-person administration by a trained assessor for 30-45 minutes, which restricts monitoring frequency and precludes physicians from adapting rehabilitation protocols to the progress of each patient. The COBRA score, computed automatically in under one minute, is shown to be strongly correlated with the FMA on an independent test cohort for two different data modalities: wearable sensors (ρ = 0.814, 95% CI [0.700,0.888]) and video (ρ = 0.736, 95% C.I [0.584, 0.838]). To demonstrate the generalizability of the approach to other conditions, the COBRA score was also applied to quantify severity of knee osteoarthritis from magnetic-resonance imaging scans, again achieving significant correlation with an independent clinical assessment (ρ = 0.644, 95% C.I [0.585,0.696]).
- Robust Anomaly Detection for Particle Physics Using Multi-background Representation LearningAbhijith Gandrakota, Lily H. Zhang, Aahlad Puli, Kyle Cranmer, Jennifer Ngadiuba, Rajesh Ranganath, and Nhan TranMLST 2024
Anomaly, or out-of-distribution, detection is a promising tool for aiding discoveries of new particles or processes in particle physics. In this work, we identify and address two overlooked opportunities to improve anomaly detection for high-energy physics. First, rather than train a generative model on the single most dominant background process, we build detection algorithms using representation learning from multiple background types, thus taking advantage of more information to improve estimation of what is relevant for detection. Second, we generalize decorrelation to the multi-background setting, thus directly enforcing a more complete definition of robustness for anomaly detection. We demonstrate the benefit of the proposed robust multi-background anomaly detection algorithms on a high-dimensional dataset of particle decays at the Large Hadron Collider.
- Robustness to Spurious Correlations Improves Semantic Out-of-Distribution DetectionLily H. Zhang, and Rajesh RanganathAAAI 2023
Methods which utilize the outputs or feature representations of predictive models have emerged as promising approaches for out-of-distribution (OOD) detection of image inputs. However, these methods struggle to detect OOD inputs that share nuisance values (e.g. background) with in-distribution inputs. The detection of shared-nuisance out-of-distribution (SN-OOD) inputs is particularly relevant in real-world applications, as anomalies and in-distribution inputs tend to be captured in the same settings during deployment. In this work, we provide a possible explanation for SN-OOD detection failures and propose nuisance-aware OOD detection to address them. Nuisance-aware OOD detection substitutes a classifier trained via empirical risk minimization and cross-entropy loss with one that 1. is trained under a distribution where the nuisance-label relationship is broken and 2. yields representations that are independent of the nuisance under this distribution, both marginally and conditioned on the label. We can train a classifier to achieve these objectives using Nuisance-Randomized Distillation (NuRD), an algorithm developed for OOD generalization under spurious correlations. Output- and feature-based nuisance-aware OOD detection perform substantially better than their original counterparts, succeeding even when detection based on domain generalization algorithms fails to improve performance.
- Understanding Failures in Out-of-distribution Detection with Deep Generative ModelsLily H. Zhang, Mark Goldstein, and Rajesh RanganathICML 2021
Deep generative models (DGMs) seem a natural fit for detecting out-of-distribution (OOD) inputs, but such models have been shown to assign higher probabilities or densities to OOD images than images from the training distribution. In this work, we explain why this behavior should be attributed to model misestimation. We first prove that no method can guarantee performance beyond random chance without assumptions on which out-distributions are relevant. We then interrogate the typical set hypothesis, the claim that relevant out-distributions can lie in high likelihood regions of the data distribution, and that OOD detection should be defined based on the data distribution’s typical set. We highlight the consequences implied by assuming support overlap between in- and out-distributions, as well as the arbitrariness of the typical set for OOD detection. Our results suggest that estimation error is a more plausible explanation than the misalignment between likelihood-based OOD detection and out-distributions of interest, and we illustrate how even minimal estimation error can lead to OOD detection failures, yielding implications for future work in deep generative modeling and OOD detection.
probabilistic models and inference
- Holdout predictive checks for Bayesian model criticismGemma E Moran, David M Blei, and Rajesh RanganathJournal of the Royal Statistical Society Series B: Statistical Methodology 2024
Bayesian modelling helps applied researchers to articulate assumptions about their data and develop models tailored for specific applications. Thanks to good methods for approximate posterior inference, researchers can now easily build, use, and revise complicated Bayesian models for large and rich data. These capabilities, however, bring into focus the problem of model criticism. Researchers need tools to diagnose the fitness of their models, to understand where they fall short, and to guide their revision. In this paper, we develop a new method for Bayesian model criticism, the holdout predictive check (HPC). Holdout predictive check are built on posterior predictive check (PPC), a seminal method that checks a model by assessing the posterior predictive distribution on the observed data. However, PPC use the data twice—both to calculate the posterior predictive and to evaluate it—which can lead to uncalibrated p-values. Holdout predictive check, in contrast, compare the posterior predictive distribution to a draw from the population distribution, a heldout dataset. This method blends Bayesian modelling with frequentist assessment. Unlike the PPC, we prove that the HPC is properly calibrated. Empirically, we study HPC on classical regression, a hierarchical model of text data, and factor analysis.
- Where to diffuse, how to diffuse, and how to get back: Automated learning for multivariate diffusionsRaghav Singhal, Mark Goldstein, and Rajesh RanganathICLR 2023
Diffusion-based generative models (DBGMs) perturb data to a target noise distribution and reverse this process to generate samples. The choice of noising process, or inference diffusion process, affects both likelihoods and sample quality. For example, extending the inference process with auxiliary variables leads to improved sample quality. While there are many such multivariate diffusions to explore, each new one requires significant model-specific analysis, hindering rapid prototyping and evaluation. In this work, we study Multivariate Diffusion Models (MDMs). For any number of auxiliary variables, we provide a recipe for maximizing a lower-bound on the MDMs likelihood without requiring any model-specific analysis. We then demonstrate how to parameterize the diffusion for a specified target noise distribution; these two points together enable optimizing the inference diffusion process. Optimizing the diffusion expands easy experimentation from just a few well-known processes to an automatic search over all linear diffusions. To demonstrate these ideas, we introduce two new specific diffusions as well as learn a diffusion process on the MNIST, CIFAR10, and ImageNet32 datasets. We show learned MDMs match or surpass bits-per-dims (BPDs) relative to fixed choices of diffusions for a given dataset and model architecture.
- Probabilistic machine learning for healthcareIrene Y Chen, Shalmali Joshi, Marzyeh Ghassemi, and Rajesh RanganathAnnual review of biomedical data science 2021
Machine learning can be used to make sense of healthcare data. Probabilistic machine learning models help provide a complete picture of observed data in healthcare. In this review, we examine how probabilistic machine learning can advance healthcare. We consider challenges in the predictive model building pipeline where probabilistic models can be beneficial including calibration and missing data. Beyond predictive models, we also investigate the utility of probabilistic machine learning models in phenotyping, in generative models for clinical use cases, and in reinforcement learning.
- Hierarchical variational modelsRajesh Ranganath, Dustin Tran, and David BleiICML 2016
Black box variational inference allows researchers to easily prototype and evaluate an array of models. Recent advances allow such algorithms to scale to high dimensions. However, a central question remains: How to specify an expressive variational distribution that maintains efficient computation? To address this, we develop hierarchical variational models (HVMs). HVMs augment a variational approximation with a prior on its parameters, which allows it to capture complex structure for both discrete and continuous latent variables. The algorithm we develop is black box, can be used for any HVM, and has the same computational efficiency as the original approximation. We study HVMs on a variety of deep discrete latent variable models. HVMs generalize other expressive variational distributions and maintains higher fidelity to the posterior.
- Black box variational inferenceRajesh Ranganath, Sean Gerrish, and David BleiAISTATS 2014 (test of time award)
test of time award
Variational inference has become a widely used method to approximate posteriors in complex latent variables models. However, deriving a variational inference algorithm generally requires significant model-specific analysis. These efforts can hinder and deter us from quickly developing and exploring a variety of models for a problem at hand. In this paper, we present a “black box” variational inference algorithm, one that can be quickly applied to many models with little additional derivation. Our method is based on a stochastic optimization of the variational objective where the noisy gradient is computed from Monte Carlo samples from the variational distribution. We develop a number of methods to reduce the variance of the gradient, always maintaining the criterion that we want to avoid difficult model-based derivations. We evaluate our method against the corresponding black box sampling based methods. We find that our method reaches better predictive likelihoods much faster than sampling methods. Finally, we demonstrate that Black Box Variational Inference lets us easily explore a wide space of models by quickly constructing and evaluating several models of longitudinal healthcare data.
robustness and generalization
- Nuisances via Negativa: Adjusting for Spurious Correlations via Data AugmentationAahlad Manas Puli, Nitish Joshi, Yoav Wald, He He, and Rajesh RanganathTransactions on Machine Learning Research 2024
In prediction tasks, there exist features that are related to the label in the same way across different settings for that task; these are semantic features or semantics. Features with vary- ing relationships to the label are nuisances. For example, in detecting cows from natural images, the shape of the head is semantic, but because images of cows often have grass back- grounds but not always, the background is a nuisance. Models that exploit nuisance-label relationships face performance degradation when these relationships change. Building mod- els robust to such changes requires additional knowledge beyond samples of the features and labels. For example, existing work uses annotations of nuisances or assumes erm-trained models depend on nuisances. Approaches to integrate new kinds of additional knowledge enlarge the settings where robust models can be built. We develop an approach to use knowledge about the semantics via data augmentations. These data augmentations cor- rupt semantic information to produce models that identify and adjust for where nuisances drive predictions. We study semantic corruptions in powering different spurious-correlation- avoiding methods on multiple out-of-distribution (ood) tasks like classifying waterbirds, natural language inference (nli), and detecting cardiomegaly in chest X-rays.
- Don’t blame dataset shift! shortcut learning due to gradients and cross entropyAahlad Manas Puli, Lily Zhang, Yoav Wald, and Rajesh RanganathAdvances in Neural Information Processing Systems 2023
Common explanations for shortcut learning assume that the shortcut improves prediction only under the training distribution. Thus, models trained in the typical way by minimizing log-loss using gradient descent, which we call default-ERM, should utilize the shortcut. However, even when the stable feature determines the label in the training distribution and the shortcut does not provide any additional information, like in perception tasks, default-ERM exhibits shortcut learning. Why are such solutions preferred when the loss can be driven to zero when using the stable feature alone? By studying a linear perception task, we show that default-ERM’s preference for maximizing the margin, even without overparameterization, leads to models that depend more on the shortcut than the stable feature. This insight suggests that default-ERM’s implicit inductive bias towards max-margin may be unsuitable for perception tasks. Instead, we consider inductive biases toward uniform margins. We show that uniform margins guarantee sole dependence on the perfect stable feature in the linear perception task and suggest alternative loss functions, termed margin control (MARG-CTRL), that encourage uniform-margin solutions. MARG-CTRL techniques mitigate shortcut learning on a variety of vision and language tasks, showing that changing inductive biases can remove the need for complicated shortcut-mitigating methods in perception tasks.
- When more is less: Incorporating additional datasets can hurt performance by introducing spurious correlationsRhys Compton, Lily Zhang, Aahlad Puli, and Rajesh RanganathMLHC 2023
In machine learning, incorporating more data is often seen as a reliable strategy for improving model performance; this work challenges that notion by demonstrating that the addition of external datasets in many cases can hurt the resulting model’s performance. In a large-scale empirical study across combinations of four different open-source chest x-ray datasets and 9 different labels, we demonstrate that in 43% of settings, a model trained on data from two hospitals has poorer worst group accuracy over both hospitals than a model trained on just a single hospital’s data. This surprising result occurs even though the added hospital makes the training distribution more similar to the test distribution. We explain that this phenomenon arises from the spurious correlation that emerges between the disease and hospital, due to hospital-specific image artifacts. We highlight the trade-off one encounters when training on multiple datasets, between the obvious benefit of additional data and insidious cost of the introduced spurious correlation. In some cases, balancing the dataset can remove the spurious correlation and improve performance, but it is not always an effective strategy. We contextualize our results within the literature on spurious correlations to help explain these outcomes. Our experiments underscore the importance of exercising caution when selecting training data for machine learning models, especially in settings where there is a risk of spurious correlations such as with medical imaging. The risks outlined highlight the need for careful data selection and model evaluation in future research and practice.
- Beyond Distribution Shift: Spurious Features Through the Lens of Training DynamicsNihal Murali, Aahlad Manas Puli, Ke Yu, Rajesh Ranganath, and othersTransactions on Machine Learning Research 2023
Deep Neural Networks (DNNs) are prone to learning spurious features that correlate with the label during training but are irrelevant to the learning problem. This hurts model generalization and poses problems when deploying them in safety-critical applications. This paper aims to better understand the effects of spurious features through the lens of the learning dynamics of the internal neurons during the training process. We make the following observations: (1) While previous works highlight the harmful effects of spurious features on the generalization ability of DNNs, we emphasize that not all spurious features are harmful. Spurious features can be "benign" or "harmful" depending on whether they are "harder" or "easier" to learn than the core features for a given model. This definition is model and dataset dependent. (2) We build upon this premise and use instance difficulty methods (like Prediction Depth) to quantify "easiness" for a given model and to identify this behavior during the training phase. (3) We empirically show that the harmful spurious features can be detected by observing the learning dynamics of the DNN’s early layers. In other words, easy features learned by the initial layers of a DNN early during the training can (potentially) hurt model generalization. We verify our claims on medical and vision datasets, both simulated and real, and justify the empirical success of our hypothesis by showing the theoretical connections between Prediction Depth and information-theoretic concepts like -usable information. Lastly, our experiments show that monitoring only accuracy during training (as is common in machine learning pipelines) is insufficient to detect spurious features. We, therefore, highlight the need for monitoring early training dynamics using suitable instance difficulty metrics.
- Out-of-distribution Generalization in the Presence of Nuisance-Induced Spurious CorrelationsAahlad Manas Puli, Lily H Zhang, Eric Karl Oermann, and Rajesh RanganathICLR 2022 2021
In many prediction problems, spurious correlations are induced by a changing relationship between the label and a nuisance variable that is also correlated with the covariates. For example, in classifying animals in natural images, the background, which is a nuisance, can predict the type of animal. This nuisance-label relationship does not always hold, and the performance of a model trained under one such relationship may be poor on data with a different nuisance-label relationship. To build predictive models that perform well regardless of the nuisance-label relationship, we develop Nuisance-Randomized Distillation (NURD). We introduce the nuisance-randomized distribution, a distribution where the nuisance and the label are independent. Under this distribution, we define the set of representations such that conditioning on any member, the nuisance and the label remain independent. We prove that the representations in this set always perform better than chance, while representations outside of this set may not. NURD finds a representation from this set that is most informative of the label under the nuisance-randomized distribution, and we prove that this representation achieves the highest performance regardless of the nuisance-label relationship. We evaluate NURD on several tasks including chest X-ray classification where, using non-lung patches as the nuisance, NURD produces models that predict pneumonia under strong spurious correlations.
- Learning invariant representations with missing dataMark Goldstein, Jörn-Henrik Jacobsen, Olina Chau, Adriel Saporta, Aahlad Manas Puli, Rajesh Ranganath, and Andrew MillerConference on Causal Learning and Reasoning 2022
Spurious correlations allow flexible models to predict well during training but poorly on related test distributions. Recent work has shown that models that satisfy particular independencies involving correlation-inducing nuisance variables have guarantees on their test performance. Enforcing such independencies requires nuisances to be observed during training. However, nuisances, such as demographics or image background labels, are often missing. Enforcing independence on just the observed data does not imply independence on the entire population. Here we derive MMD estimators used for invariance objectives under missing nuisances. On simulations and clinical data, optimizing through these estimates achieves test performance similar to using estimators that make use of the full data.
survival analysis
- Development and external validation of a dynamic risk score for early prediction of cardiogenic shock in cardiac intensive care units using machine learningYuxuan Hu, Albert Lui, Mark Goldstein, Mukund Sudarshan, Andrea Tinsay, Cindy Tsui, Samuel D Maidman, John Medamana, Neil Jethani, Aahlad Puli, and othersEuropean Heart Journal: Acute Cardiovascular Care 2024
Myocardial infarction and heart failure are major cardiovascular diseases that affect millions of people in the USA with morbidity and mortality being highest among patients who develop cardiogenic shock. Early recognition of cardiogenic shock allows prompt implementation of treatment measures. Our objective is to develop a new dynamic risk score, called CShock, to improve early detection of cardiogenic shock in the cardiac intensive care unit (ICU).
- An effective meaningful way to evaluate survival modelsShi-ang Qi, Neeraj Kumar, Mahtab Farrokh, Weijie Sun, Li-Hao Kuan, Rajesh Ranganath, Ricardo Henao, and Russell GreinerICML 2023
One straightforward metric to evaluate a survival prediction model is based on the Mean Absolute Error (MAE) – the average of the absolute difference between the time predicted by the model and the true event time, over all subjects. Unfortunately, this is challenging because, in practice, the test set includes (right) censored individuals, meaning we do not know when a censored individual actually experienced the event. In this paper, we explore various metrics to estimate MAE for survival datasets that include (many) censored individuals. Moreover, we introduce a novel and effective approach for generating realistic semi-synthetic survival datasets to facilitate the evaluation of metrics. Our findings, based on the analysis of the semi-synthetic datasets, reveal that our proposed metric (MAE using pseudo-observations) is able to rank models accurately based on their performance, and often closely matches the true MAE – in particular, is better than several alternative methods.
- Survival mixture density networksXintian Han, Mark Goldstein, and Rajesh RanganathMLHC 2022
Survival analysis, the art of time-to-event modeling, plays an important role in clinical treatment decisions. Recently, continuous time models built from neural ODEs have been proposed for survival analysis. However, the training of neural ODEs is slow due to the high computational complexity of neural ODE solvers. Here, we propose an efficient alternative for flexible continuous time models, called Survival Mixture Density Networks (Survival MDNs). Survival MDN applies an invertible positive function to the output of Mixture Density Networks (MDNs). While MDNs produce flexible real-valued distributions, the invertible positive function maps the model into the time-domain while preserving a tractable density. Using four datasets, we show that Survival MDN performs better than, or similarly to continuous and discrete time baselines on concordance, integrated Brier score and integrated binomial log-likelihood. Meanwhile, Survival MDNs are also faster than ODE-based models and circumvent binning issues in discrete models.
- Inverse-weighted survival gamesXintian Han, Mark Goldstein, Aahlad Puli, Thomas Wies, Adler Perotte, and Rajesh RanganathNeurIPS 2021
Deep models trained through maximum likelihood have achieved state-of-the-art results for survival analysis. Despite this training scheme, practitioners evaluate models under other criteria, such as binary classification losses at a chosen set of time horizons, e.g. Brier score (BS) and Bernoulli log likelihood (BLL). Models trained with maximum likelihood may have poor BS or BLL since maximum likelihood does not directly optimize these criteria. Directly optimizing criteria like BS requires inverse-weighting by the censoring distribution. However, estimating the censoring model under these metrics requires inverse-weighting by the failure distribution. The objective for each model requires the other, but neither are known. To resolve this dilemma, we introduce Inverse-Weighted Survival Games. In these games, objectives for each model are built from re-weighted estimates featuring the other model, where the latter is held fixed during training. When the loss is proper, we show that the games always have the true failure and censoring distributions as a stationary point. This means models in the game do not leave the correct distributions once reached. We construct one case where this stationary point is unique. We show that these games optimize BS on simulations and then apply these principles on real world cancer and critically-ill patient data.
- X-cal: Explicit calibration for survival analysisMark Goldstein, Xintian Han, Aahlad Puli, Adler Perotte, and Rajesh RanganathNeurIPS 2020
Survival analysis models the distribution of time until an event of interest, such as discharge from the hospital or admission to the ICU. When a model’s predicted number of events within any time interval is similar to the observed number, it is called well-calibrated. A survival model’s calibration can be measured using, for instance, distributional calibration (D-CALIBRATION) (Haider et al., 2020) which computes the squared difference between the observed and predicted number of events within different time intervals. Classically, calibration is addressed in post-training analysis. We develop explicit calibration (X-CAL), which turns DCALIBRATION into a differentiable objective that can be used in survival modeling alongside maximum likelihood estimation and other objectives. X-CAL allows practitioners to directly optimize calibration and strike a desired balance between predictive power and calibration. In our experiments, we fit a variety of shallow and deep models on simulated data, a survival dataset based on MNIST, on lengthof-stay prediction using MIMIC-III data, and on brain cancer data from The Cancer Genome Atlas. We show that the models we study can be miscalibrated. We give experimental evidence on these datasets that X-CAL improves D-CALIBRATION without a large decrease in concordance or likelihood.
- Deep survival analysis: Nonparametrics and missingnessXenia Miscouridou, Adler Perotte, Noémie Elhadad, and Rajesh RanganathMLHC 2018
Clinical care requires understanding the time to medical events. Medical events include the time to a disease like chronic kidney disease progressing or the time to a complication as in stroke for high blood pressure. Models for event times live in the framework provided by survival analysis. We expand on deep survival analysis, a deep generative model for survival analysis in the presence of missing data, where the survival times are modeled using Weibull distributions. We develop methods to relax the distributional assumptions in deep survival analysis using survival distributions that can approximate any true survival function. We show that the model structure mimics the information-optimal procedure in the presence of missing data. Our experiments demonstrate that moving to flexible survival functions yields better likelihoods and concordances for coronary heart disease prediction from electronic health records.
- Deep survival analysisRajesh Ranganath, Adler Perotte, Noémie Elhadad, and David BleiMLHC 2016
The electronic health record (EHR) provides an unprecedented opportunity to build actionable tools to support physicians at the point of care. In this paper, we introduce deep survival analysis, a hierarchical generative approach to survival analysis in the context of the EHR. It departs from previous approaches in two main ways: (1) all observations, including covariates, are modeled jointly conditioned on a rich latent structure; and (2) the observations are aligned by their failure time, rather than by an arbitrary time zero as in traditional survival analysis. Further, it handles heterogeneous data types that occur in the EHR. We validate deep survival analysis by stratifying patients according to risk of developing coronary heart disease (CHD) on 313,000 patients corresponding to 5.5 million months of observations. When compared to the clinically validated Framingham CHD risk score, deep survival analysis is superior in stratifying patients according to their risk.
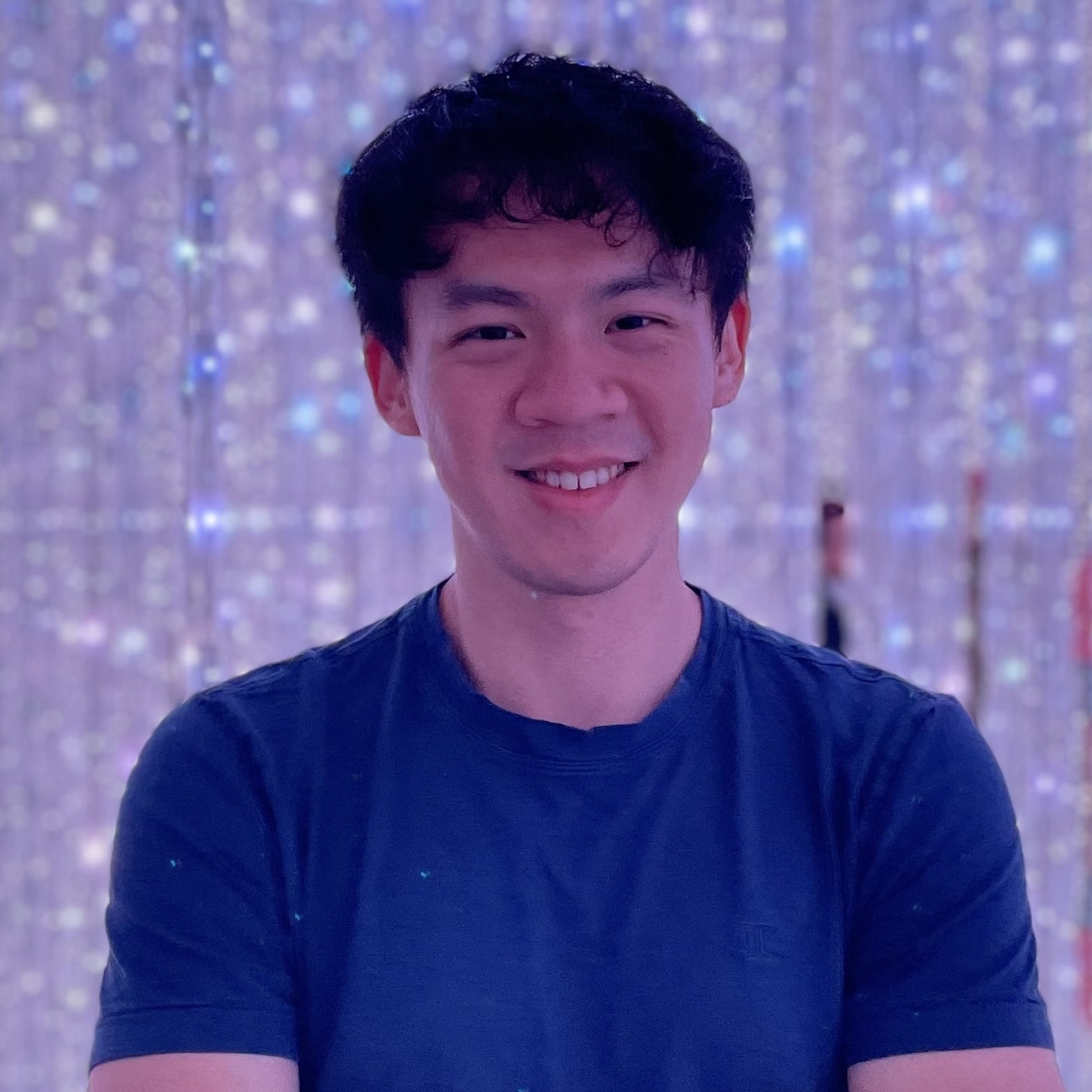
Wanqian Yang
PhD Student
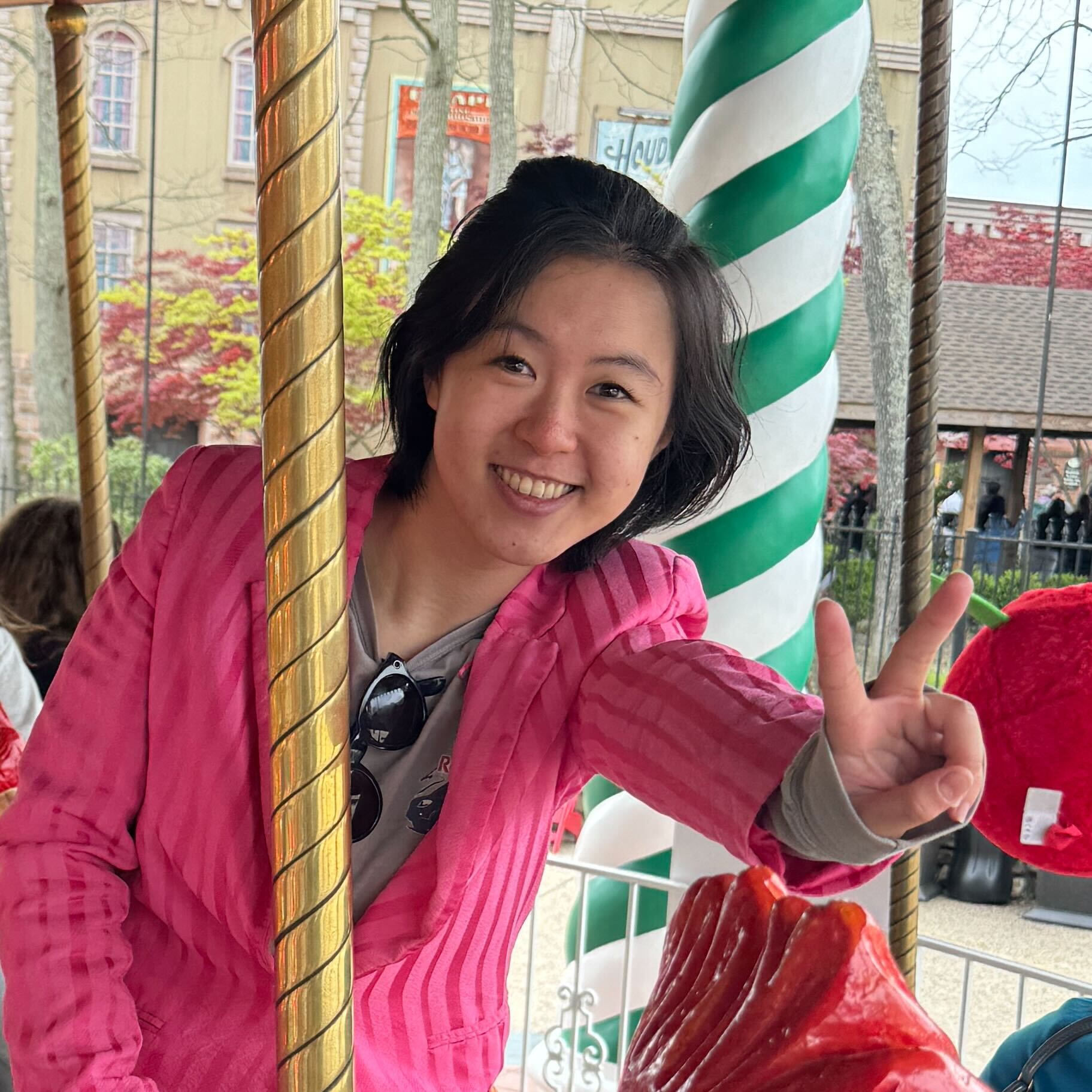
Boyang Yu
PhD Student
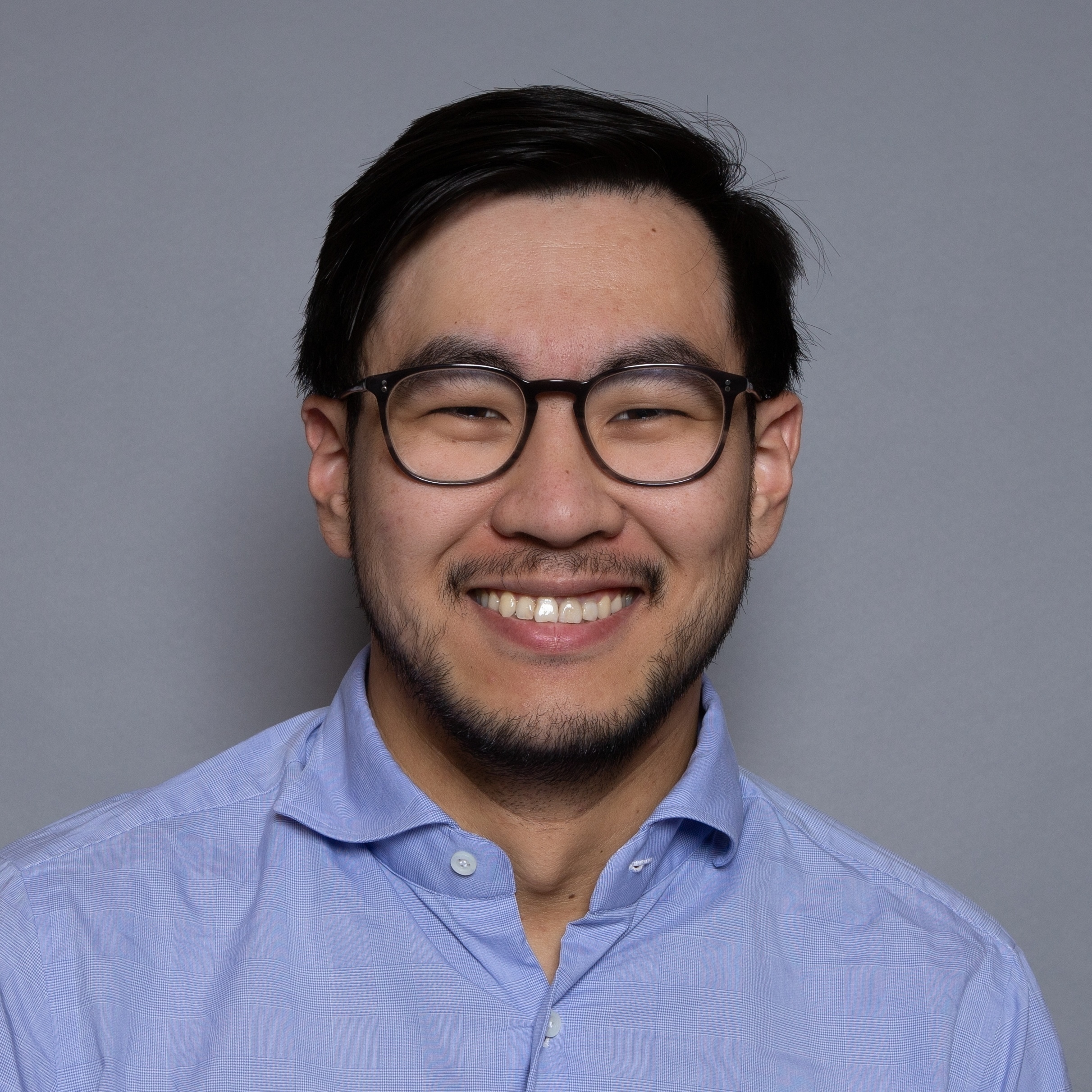
Hao Zhang
PhD Student
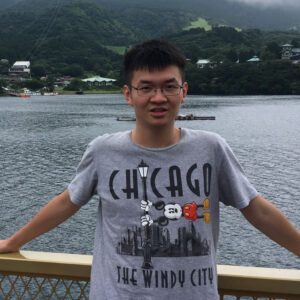
Xintian Han
PhD Student
Courant Institute of Mathematical Sciences
New York University
60 Fifth Avenue
New York, NY 10011
rajeshr at cims dot nyu dot edu